A human eye?
Artificial intelligence is already having a significant impact on healthcare, including in radiography. Synergy asks the experts to learn how the technology may impact the profession
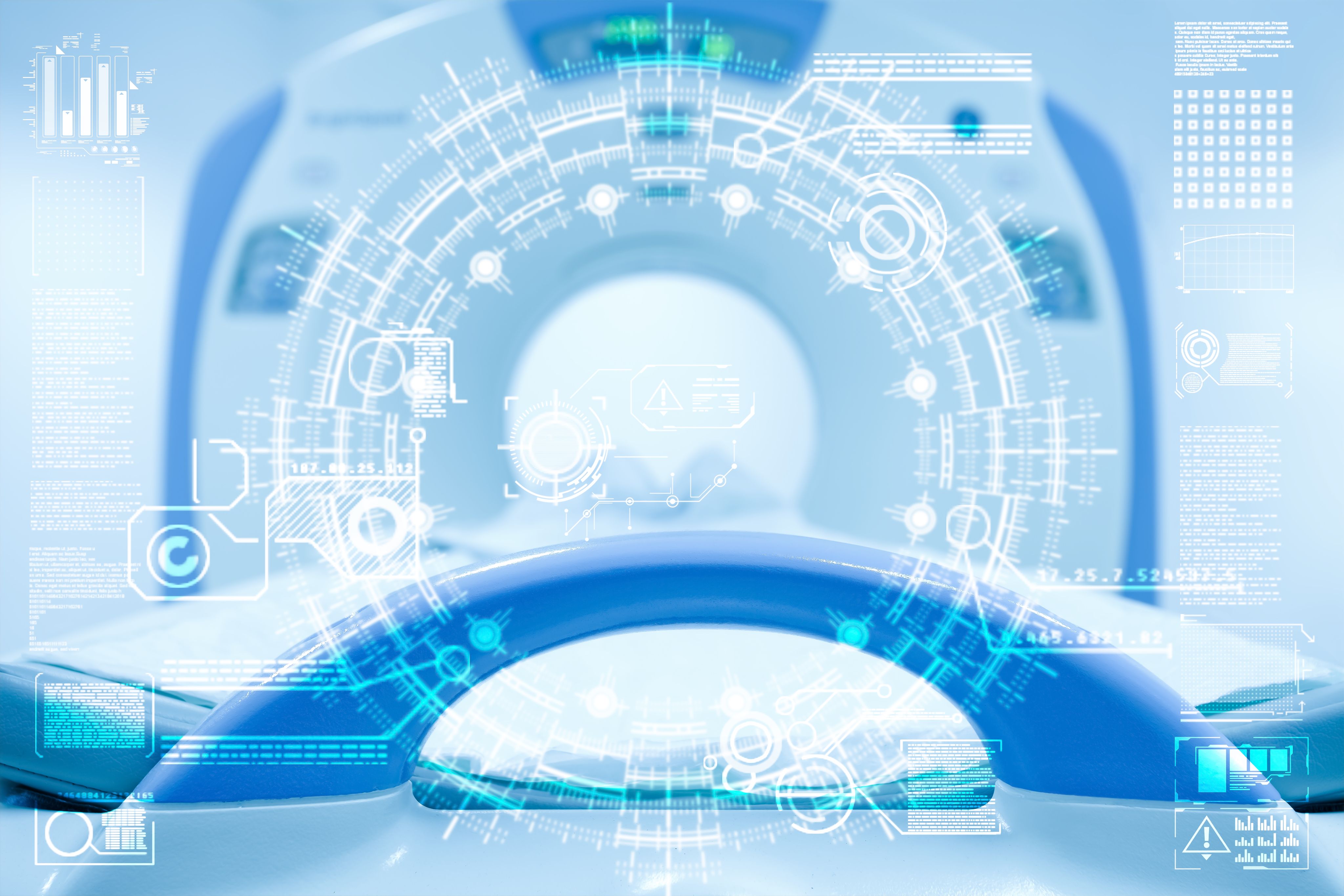
A human eye?
Artificial intelligence is already having a significant impact on healthcare, including in radiography. Synergy sits down with the experts to learn how the technology may impact the profession
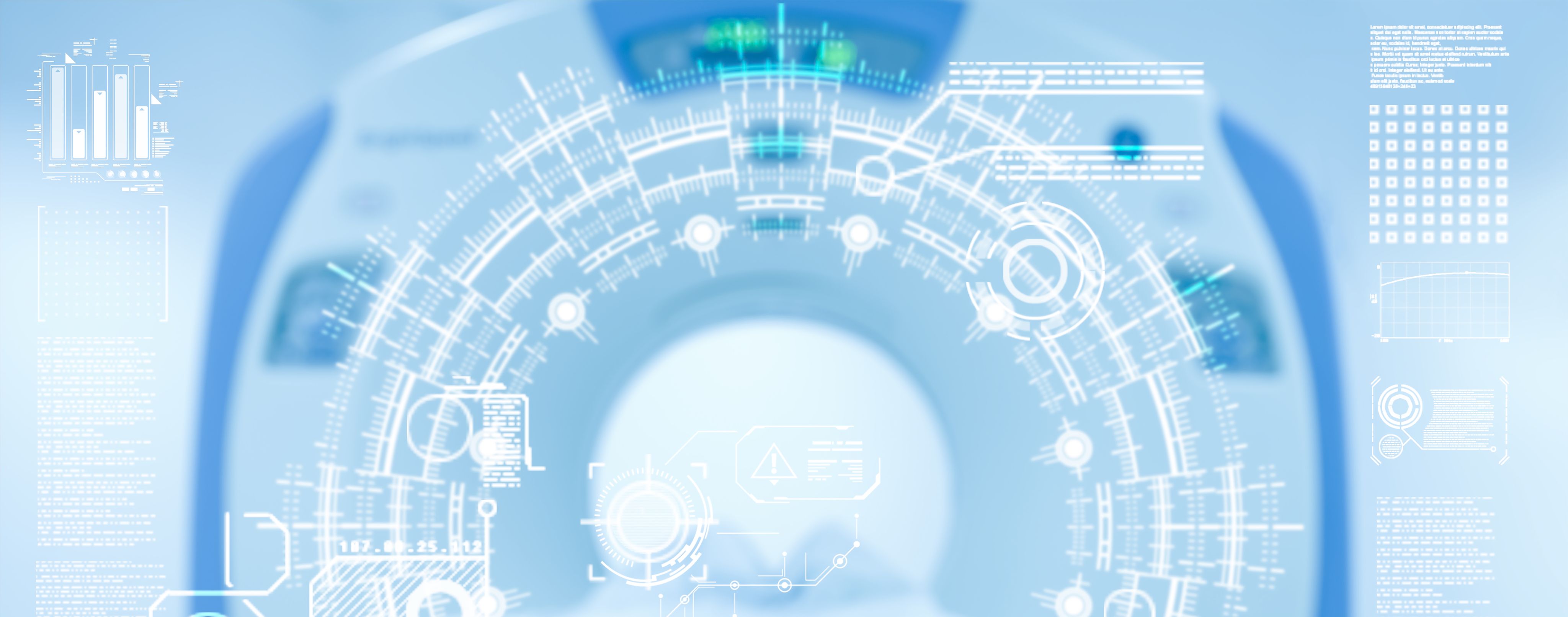
Artificial intelligence has been granted a newfound focus in recent years, as rapid advancements in generative AI raise questions about how it can be used across professional fields.
But there is more to AI than responding to questions or creating images – its potential impacts on radiography cannot be underestimated.
AI is already being used across radiography in a variety of ways. In imaging, the technology can help ensure effective scanning by tracking patient’s body movements to keep them aligned, by accurately judging the amount of radiation incurred during radiotherapy to reduce dosage, and by aiding radiographers in diagnosing scans.
For administrative work, AI is helping with dictation and note-keeping, with appointment scheduling and forecasting and by ensuring effective and efficient staff assignment with e-rostering.
The NHS England Digital Framework for Allied Health Professionals recognises that digital health solutions can improve quality, safety and efficiency of patient care.
It explains: “Compared to other AHPs, radiographers will increasingly be exposed to some of the more advanced developments in digital diagnostic capabilities through the evolution of artificial intelligence and machine learning innovation in clinical decision support.”
In 2022, Unity Insights and Health Education England published a study which found 240 different AI technologies had been deployed in the NHS. 34 per cent of those technologies were being deployed in diagnostics, and of those, just under half (49 per cent) were involved with imaging solutions.
This roadmap identified the top workforce groups with the most potential to use AI (by percentage of applicable technologies) as:
Artificial intelligence has been granted a newfound focus in recent years, as rapid advancements in generative AI raise questions about how it can be used across professional fields. But there is more to AI than responding to questions or creating images – its potential to improve the efficiency, effectiveness and quality of radiography cannot be underestimated.
The NHS England Framework for Allied Health Professionals recognises that digital health solutions can improve quality, safety and efficiency.
It explains: “Compared to other AHPs, radiographers will increasingly be exposed to some of the more advanced developments in digital diagnostic capabilities through the evolution of artificial intelligence and machine learning innovation in clinical decision support.”
In 2022, meanwhile, Unity Insights and Health Education England published a study which found 240 different AI technologies had been deployed in the NHS. Of these 240 technologies, 34 per cent were being deployed in diagnostics, and of that 34 per cent, just under half (49 per cent) were involved with imaging solutions.
This roadmap identified the top workforce groups with the most potential to use AI (by percentage of applicable technologies) as:
Clinical input
Dr. Tracy O’Regan, professional officer of clinical imaging and research at the SoR, spoke to Synergy to explain how radiographers currently use AI.
Tracy explained that various forms of AI - such as deep learning, machine learning and neural networks - are being deployed across radiography.
“Discussion around deep learning and neural networks is mostly related to image perception,” she said.
“But if you’re talking about back office and workflow use, then the discussion of AI tends to be around the use of less complex computer algorithms designed to complete a task that would traditionally have been carried out by a human.”
“This is not new for us,” Tracy emphasised. “We’ve been digital for a long time in radiology and radiotherapy, for example with digital imaging, use of voice recognition for reporting, and picture archiving communications. At some trusts, patient self check-in has been going on for quite a while now. We are digitally enabled, probably because of the ways we work within radiography, therapeutics and clinical imaging. It’s already happened.”
Generative AI such as ChatGPT, meanwhile, presented different and more difficult use cases. The technology could, for example, be used to generate patient leaflets or physiology treatment programmes.
However, it also presents problems.
“Generative AI is a concern in terms of learning,” Tracy said. “Production of essays, for example – it’s a concern over whether people are still going to get that quality of learning. Our lecturers and practice educators remain pivotal to supporting students' development of evidence-based practice and critical thinking skills.”
Dr Tracey O'Regan
Dr Tracey O'Regan
Clinical input
Tracey O'Regan
Tracey O'Regan
Dr. Tracy O’Regan, professional officer of clinical imaging and research at the SoR, spoke to Synergy to explain how radiographers currently use AI.
Tracy explained that various forms of AI - such as deep learning, machine learning and neural networks - are being deployed across radiography.
“Discussion around deep learning and neural networks is mostly related to image perception,” she said.
“But if you’re talking about back office and workflow use, then the discussion of AI tends to be around the use of less complex computer algorithms designed to complete a task that would traditionally have been carried out by a human.”
“This is not new for us,” Tracy emphasised. “We’ve been digital for a long time in radiology and radiotherapy, for example with digital imaging, use of voice recognition for reporting, and picture archiving communications. At some trusts, patient self check-in has been going on for quite a while now. We are digitally enabled, probably because of the ways we work within radiography, therapeutics and clinical imaging. It’s already happened.”
Generative AI such as ChatGPT, meanwhile, presented different and more difficult use cases. The technology could, for example, be used to generate patient leaflets or physiology treatment programmes.
However, it also presents problems.
“Generative AI is a concern in terms of learning,” Tracy said. “Production of essays, for example – it’s a concern over whether people are still going to get that quality of learning. Our lecturers and practice educators remain pivotal to supporting students' development of evidence-based practice and critical thinking skills.”
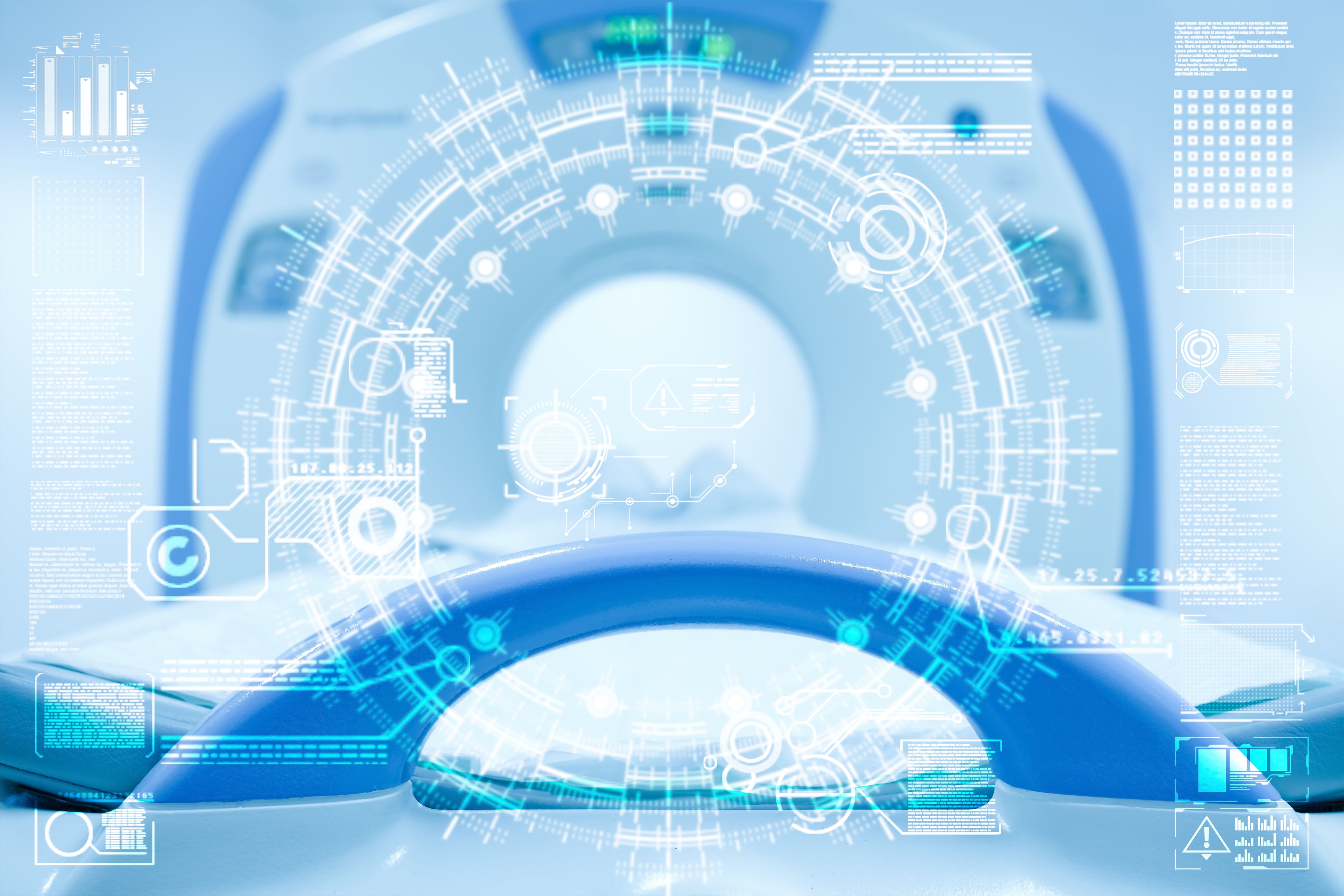
Human oversight
The Society of Radiographers has released official guidance for the past few years surrounding the importance of implementing AI technology in a safe, efficient and effective way.
It warns: “AI is being rapidly integrated into imaging equipment with arguably little consideration as to how it influences radiography practice and frontline services. Current regulatory frameworks stipulate that all AI systems that are deployed clinically require human oversight of their implementation.
“This is potentially adding to the radiographer’s role and increasing the need for a high level of digital fluency among radiography staff as they must learn to evaluate, interact and oversee the actions of AI driven tools within their workflow.”
The Society calls for research to investigate the impact of AI on: quality of service, patient care and radiographers’ roles and working practices.
It adds there is a need for ‘real world’ clinical validation of AI interventions.
Tracy reiterated that while development of many different kinds of AI technologies remained ongoing, the principles of the SoR policy statement on AI are an important strategy. The SoR aims to influence and direct improvements that can be made by implementing new technology in healthcare, locally, nationally, and internationally, and to promote safe and ethical practice that will enable better patient service user outcomes.
She said: “It’s got to be safe and effective, and also consider if it is economically effective? What is it we need to assist clinicians and patients?”
The Society’s AI Advisory Group has also released a series of webinars, policy and guidance documents for radiographers interested in getting to grips with the technology, its use, and its potential impacts on the profession.
Alongside policy on ensuring members are consulted at every stage, SoR guidance states:
- Radiographers should will take into account the opportunities for innovation and development of practice and patient care that are afforded by evolving technology, AI, machine learning and deep learning
- The guidance development was mindful of opportunities to adopt AI systems for the improvement of its service to the membership, and new initiatives will be tested and assessed to strike the right balance between additional efficiency with appropriate transparency and member engagement
- The protection of patient data is paramount
The guidance adds: “We would expect that as a minimum standard, any AI system will have clinical governance measures in place and will be compliant with current Quality Standards for Imaging and UK GDPR.”
Human oversight
The Society of Radiographers has released official guidance for the past few years surrounding the importance of implementing AI technology in a safe, efficient and effective way.
It warns: “AI is being rapidly integrated into imaging equipment with arguably little consideration as to how it influences radiography practice and frontline services. Current regulatory frameworks stipulate that all AI systems that are deployed clinically require human oversight of their implementation.
“This is potentially adding to the radiographer’s role and increasing the need for a high level of digital fluency among radiography staff as they must learn to evaluate, interact and oversee the actions of AI driven tools within their workflow.”
The Society calls for research to investigate the impact of AI on: quality of service, patient care and radiographers’ roles and working practices.
It adds there is a need for ‘real world’ clinical validation of AI interventions.
Tracy reiterated that while development of many different kinds of AI technologies remained ongoing, the principles of the SoR policy statement on AI are an important strategy. The SoR aims to influence and direct improvements that can be made by implementing new technology in healthcare, locally, nationally, and internationally, and to promote safe and ethical practice that will enable better patient service user outcomes.
She said: “It’s got to be safe and effective, and also consider if it is economically effective? What is it we need to assist clinicians and patients?”
The Society’s AI Advisory Group has also released a series of webinars, policy and guidance documents for radiographers interested in getting to grips with the technology, its use, and its potential impacts on the profession.
Alongside policy on ensuring members are consulted at every stage, SoR guidance states:
- Radiographers should will take into account the opportunities for innovation and development of practice and patient care that are afforded by evolving technology, AI, machine learning and deep learning
- The guidance development was mindful of opportunities to adopt AI systems for the improvement of its service to the membership, and new initiatives will be tested and assessed to strike the right balance between additional efficiency with appropriate transparency and member engagement
- The protection of patient data is paramount
The guidance adds: “We would expect that as a minimum standard, any AI system will have clinical governance measures in place and will be compliant with current Quality Standards for Imaging and UK GDPR.”
Quality assurance
Avneet Gill is a diagnostic radiographer currently undertaking a PhD at Ulster University focusing on potential new applications of AI. She sat down with Synergy to discuss her research.
She explained Quality Assurance is one area AI can help radiographers with – many NHS Trusts now use Computer-Aided Diagnosis (CAD) alongside their imaging, which can “run in the background and wean out the easy ones,” Avneet said. This software processes images to identify typical appearances and highlight conspicuous sections.
CAD AI usage varies across the country, and is currently used mostly for pathology detection.
AI can also be used to advise on image optimisation, Avneet continued, by informing the radiographer if the patient is positioned correctly or if a scan should be repeated. A similar technology can track patient positioning and cease scanning if a patient moves out of position.
Avneet added: “Our workforce is trying loads of different things to support the lack of radiographers and apprentices. There is a shortage, but AI is a way to support that – it can improve the detection of pathologies, it can improve the amount of scans being reported. It is a positive thing.”
Avneet described a novel AI technology that sought to aid in triage, sorting scans by severity for radiographers to report: “There's so much potential for where it can go. The breadth and scope of a radiographer’s responsibilities is going to be expanded to include most modalities. The new registrants are going to need to understand everything. How are we going to do all that without help?”
Claire Currie, senior lecturer in diagnostic imaging and part time PhD student at Glasgow Caledonian University, agreed the scope of radiography is growing, and there is a need for support to meet this extra capacity.
Demand for radiology services is only growing, driven by an ageing population, changing clinical pathways, new guidelines, and increased screening to support early diagnosis.
“It is now urgent that strategies are put in place to ease this disconnect,” she added.
One way this might be achieved is through a clinical decision support (CDS) tool, which aims to eliminate inappropriate referrals and missing request information. CDS systems guide clinical decision-making, and was one of the earliest forms of AI in radiology departments.
Claire said she believes there is potential for CDS to be updated and advanced, for more personalised support.
CDS systems work by connecting signs and symptoms to diagnoses and imaging tests, as well as rules and guidelines with actual patient data, such as case symptoms and clinical history, before producing a radiology referral, and presenting the clinician with the most up-to-date rules or guidance.
“A CDS can improve the quality of patient care foremost, followed by potential workflow efficiencies as well as efficient management of resources,” Claire said.
“For the quality of care, CDS could ensure that patients receive the right test the first time, allowing or enabling clinicians to provide or take patients to the best treatment pathway and, by extension, minimising any necessary radiation to the patient.”
Quality assurance
Avneet Gill is a diagnostic radiographer currently undertaking a PhD at Ulster University focusing on potential new applications of AI. She sat down with Synergy to discuss her research.
She explained Quality Assurance is one area AI can help radiographers with – many NHS Trusts now use Computer-Aided Diagnosis (CAD) alongside their imaging, which can “run in the background and wean out the easy ones,” Avneet said. This software processes images to identify typical appearances and highlight conspicuous sections.
CAD AI usage varies across the country, and is currently used mostly for pathology detection.
AI can also be used to advise on image optimisation, Avneet continued, by informing the radiographer if the patient is positioned correctly or if a scan should be repeated. A similar technology can track patient positioning and cease scanning if a patient moves out of position.
Avneet added: “Our workforce is trying loads of different things to support the lack of radiographers and apprentices. There is a shortage, but AI is a way to support that – it can improve the detection of pathologies, it can improve the amount of scans being reported. It is a positive thing.”
Avneet described a novel AI technology that sought to aid in triage, sorting scans by severity for radiographers to report.
“There's so much potential for where it can go. The breadth and scope of a radiographer’s responsibilities is going to be expanded to include most modalities. The new registrants are going to need to understand everything. How are we going to do all that without help?”
Claire Currie, senior lecturer in diagnostic imaging and part time PhD student at Glasgow Caledonian University, agreed the scope of radiography is growing, and there is a need for support to meet this extra capacity.
Demand for radiology services is only growing, driven by an ageing population, changing clinical pathways, new guidelines, and increased screening to support early diagnosis.
“It is now urgent that strategies are put in place to ease this disconnect,” she added.
One way this might be achieved is through a clinical decision support (CDS) tool, which aims to eliminate inappropriate referrals and missing request information. CDS systems guide clinical decision-making, and was one of the earliest forms of AI in radiology departments.
Claire believes there is potential for CDS to be updated and advanced, for more personalised support.
CDS systems work by connecting signs and symptoms to diagnoses and imaging tests, as well as rules and guidelines with actual patient data, such as case symptoms and clinical history, before producing a radiology referral, and presenting the clinician with the most up-to-date rules or guidance.
“A CDS can improve the quality of patient care foremost, followed by potential workflow efficiencies as well as efficient management of resources,” Claire said.
“For the quality of care, CDS could ensure that patients receive the right test the first time, allowing or enabling clinicians to provide or take patients to the best treatment pathway and, by extension, minimising any necessary radiation to the patient.”
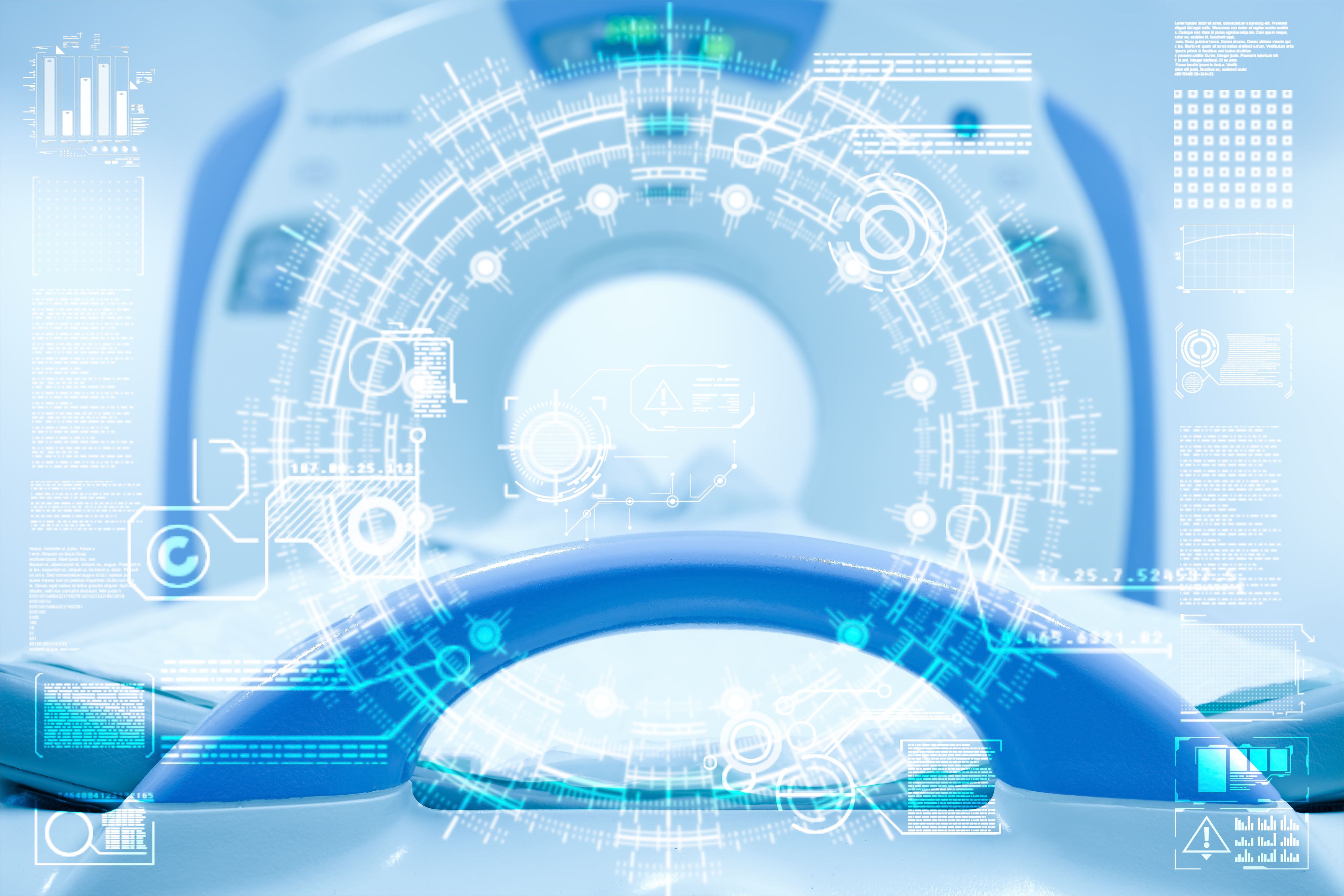
Safe and effective?
Tracy explained that questions of evaluation are important to answer now, to ensure effective implementation and governance of AI into the future.
“What will the research or service evaluation approach be? What metrics will be measured and will findings be transferrable to other sites and for other use cases? Is it safe and effective? Is it going to make a difference to the patient pathway, to patient outcomes? How is it going to affect the staff and the roles that we undertake?
Tracy described some AI use cases tackling problems that are unlikely to make a difference – AI that checks whether a knee image is reversed, for example, should have little practical impact, because radiographers are trained to check key reversal markers during image evaluation.
Avneet, meanwhile, warned people are resistant to the use of AI because of the risks of it becoming a “black box”, with the users unable to understand how it works.
Evidence also suggests people are more likely to favour advice from automated decision-making systems, even when contradictory information is provided from a non-automated source.
“People do rely on it maybe too heavily, and then they get into trouble and it leads to more of a negative perception of AI,” she said.
“There’s also some confusion from radiologists and reporting radiographers around who takes legal responsibility. If an AI algorithm says, ‘this chest X-ray has lung cancer’, and the department follows treatment for that, but it turns out that wasn't correct, then someone has to be to blame.
“That patient might have undergone extra treatment, or extra imaging, or extra radiation they didn't need. People are a bit scared of that legal aspect of it. The amount of products out there which haven't been tested on a really large dataset is quite astounding.”
Advancing and improving
Avneet emphasised that it is an assistive technology, and radiographers should not be worried about AI taking their jobs.
“It will just help you,” she insisted. “There were so many times when I worked in A&E, and it was the middle of the night, and the radiologist was doing a procedure – I couldn't ask them for help. If I just had something to back me up and suggest things, like, ‘that's definitely a pneumothorax’, it would have eased the process a little bit more. I could have conferred with the junior doctor if there was any confusion – it just makes it a little bit easier.”
Rebecca Hawkesford is a Therapeutic Radiographer at UHCW Coventry and Warwickshire and a teaching fellow at Coventry University for enhanced and return to practice. She is also a member of the SoR’s AI Advisory Group.
She said AI could aid in forecasting and scheduling departmental needs, such as staffing requirements, which could enable managers to plan for future job roles in a proactive manner – “rather than the reactive process it tends to be now,” she explained.
“This would allow managers to plan short and long term for the staffing needs of the department. It could also be used to predict appointment scheduling using yearly trends, as certain times of the year are quieter than others. This would allow departments to schedule staff training and development at these times.”
In addition to planning, AI could also aid with staff training.
She added: “Whilst the majority of departments now have practice educators, AI could assist with the training of new staff by providing off-line virtual scenarios and clinical situations for the staff to work through. This would provide trainees with a safe environment to ask questions, rather than feeling pressured in on-line situations in their preceptorship period.”
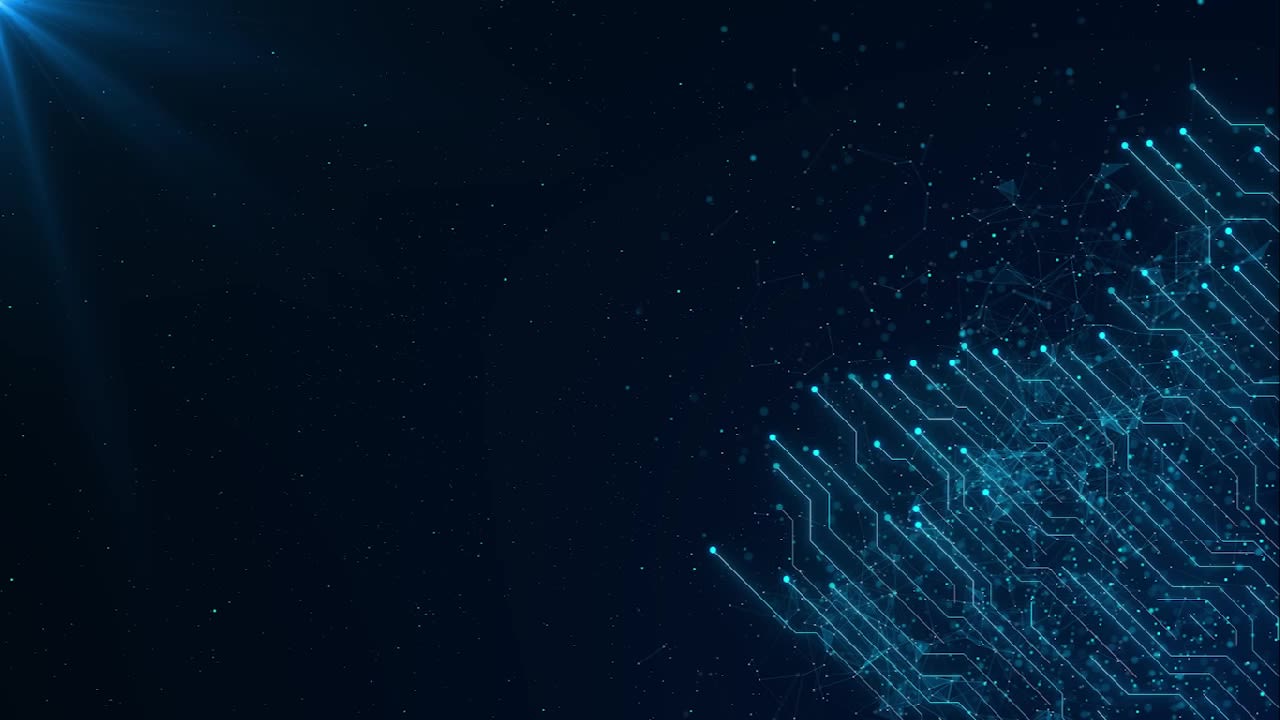
Advancing and Improving
Avneet emphasised that it is an assistive technology, and radiographers should not be worried about AI taking their jobs.
“It will just help you,” she insisted. “There were so many times when I worked in A&E, and it was the middle of the night, and the radiologist was doing a procedure – I couldn't ask them for help. If I just had something to back me up and suggest things, like, ‘that's definitely a pneumothorax’, it would have eased the process a little bit more. I could have conferred with the junior doctor if there was any confusion – it just makes it a little bit easier.”
Rebecca Hawkesford is a Therapeutic Radiographer at UHCW Coventry and Warwickshire and a teaching fellow at Coventry University for enhanced and return to practice. She is also a member of the SoR’s AI Advisory Group.
She said AI could aid in forecasting and scheduling departmental needs, such as staffing requirements, which could enable managers to plan for future job roles in a proactive manner – “rather than the reactive process it tends to be now,” she explained.
“This would allow managers to plan short and long term for the staffing needs of the department. It could also be used to predict appointment scheduling using yearly trends, as certain times of the year are quieter than others. This would allow departments to schedule staff training and development at these times.”
In addition to planning, AI could also aid with staff training.
She added: “Whilst the majority of departments now have practice educators, AI could assist with the training of new staff by providing off-line virtual scenarios and clinical situations for the staff to work through. This would provide trainees with a safe environment to ask questions, rather than feeling pressured in on-line situations in their preceptorship period.”
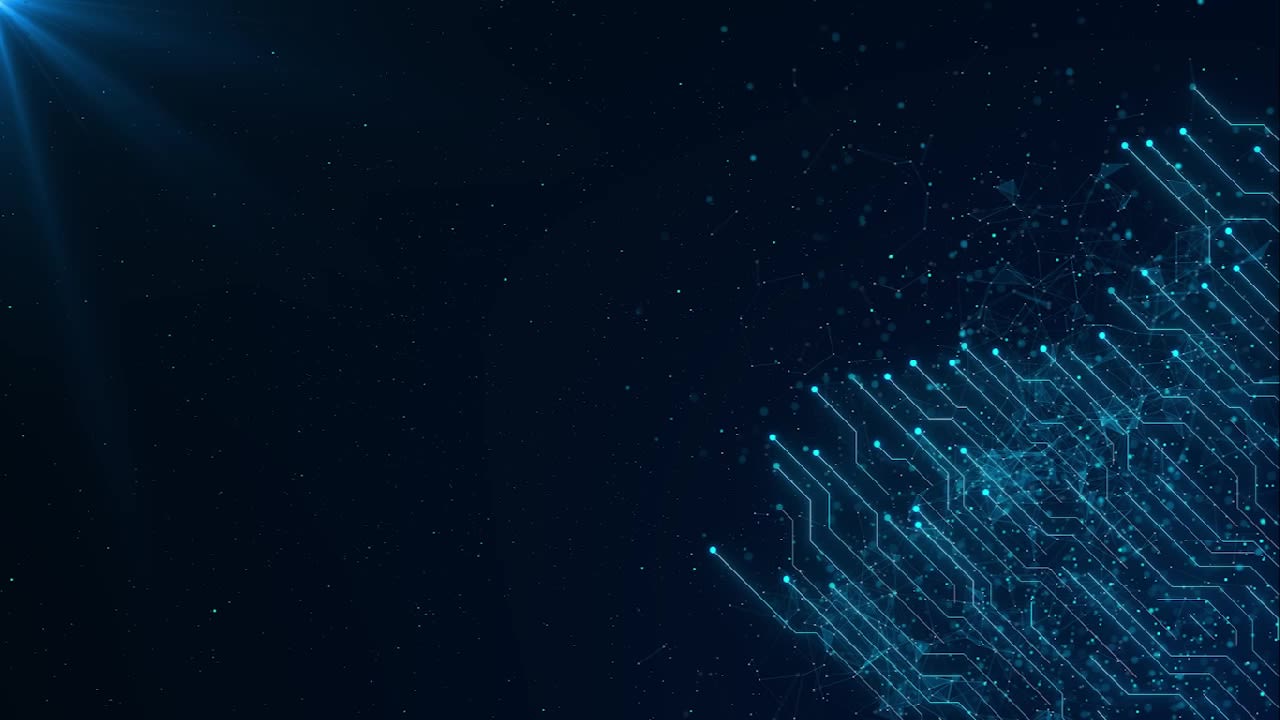
AI leadership
A blog post published on the BMJ website in November 2023, written by Gemma Walsh, Dr Christina Malamateniou, director of the postgraduate programme of radiography at City, University of London, and Dr. Janice St. John-Matthews, head of Allied Health Professions at NHS England, emphasised the impact of workforce pressures on the ability to innovate.
They wrote: “Radiographers have little time to participate in self-guided, continuous professional development in their own time. What radiographers need is a clear pathway into AI leadership that is both nationally recognised and can be supported by radiology management, giving them the time and opportunity to develop themselves. Leadership support and AI education needs to be specific to radiographers.”
Find out more...
The Society of Radiographers AI Advisory Group initially convened in September 2020, following the release of the SoR policy statement on AI.
The working group was established to represent the voice of the radiographic workforce in the development of AI technology, with aims to strengthen and support care for patients in clinical imaging and radiotherapy.
It also acts as a point of contact, and to provide clinical, educational, and researched-related radiography advice.
Find out more about the AI Advisory Group here.
You can also follow the group on X here.
Image credits: shivkantsharma07 / Creatas Video+ / Getty Images Plus
XH4D / iStock / Getty Images Plus
Now read...
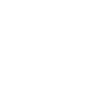