The future of ultrasound
How artificial intelligence will shape our clinical practice
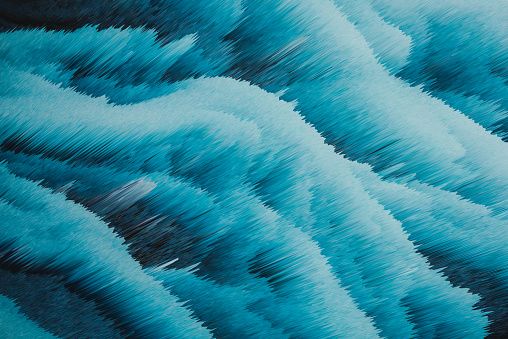
The age of artificial intelligence (AI) has arrived and is expanding rapidly. In the past decade, it has conquered a number of tasks, including agile-legged robot locomotion, recognition of objects in images, speech recognition and machine translation, with self-driving cars one of the developments likely to be perfected in the next
wave1.
In recent years, the conversation about the impending and potential impact of AI on our clinical practice within the medical imaging community has been inescapable.
But why all the hype?
Radiology as a field is no newbie to technological advances and change, and the shift to filmless and paperless departments seems a distant memory. Successive advances in ultrasound technologies have seen development from grainy B-mode images to the use of capacitive micromachined ultrasonic transducers (CMUT), which operate at the widest range of frequencies on handheld devices; to 3D and 4D technologies; ultrasonic elastography; shear wave elastography (SWE); and contrast-enhanced ultrasound (CEUS), to name but a few2. Indeed, technology and innovation, coupled with high-quality patient care and clinical services, are the foundation of what we do. So, is AI technology really the game changer that the scientists, clinical academics and industry leaders would have us believe?
Major advances in graphic and central processing units (GPU and CPUs) – along with the development of machine learning (ML) algorithms (particularly deep learning methodologies), the availability of large clinical imaging datasets and limitations in the largely qualitative methods by which images are reviewed by radiologists, radiographers or sonographers – have established AI in medical imaging as a major scientific interest2.
AI in mammography is a notable example of potential AI development. Longstanding skills and staff shortages affecting the National Breast Screening Programme have ultimately led to the development of AI tools that can support screening, with a view to reducing the resource use (read: jobs, costs, time) and the workload for staff who read mammograms. In 2021, the National Institute for Health and Care Excellence (NICE) produced guidance in the form of a Medtech Innovation Briefing on AI in mammography, reviewing the intended use, evidence, uncertainties and related costs of five technologies commercially available to the NHS. This technology is already here and we are likely to see steady expansion in radiology in the next five to 10 years3–5.
While ultrasound has not yet seen major prospective clinical trials related to AI tools, ultrasound equipment manufacturers have been quick to develop their research and development programmes to realise the potential in standalone products. For example, General Electric Healthcare, Philips Healthcare and Siemens Healthineers are among the major manufactures to have incorporated deep learning-based AI offerings – echocardiography, obstetrics, gynaecology, abdominal aortic screening, lung ultrasound and more – that are all easily purchased as add-on packages with ultrasound equipment.
Despite the consistent growth in demand for ultrasound examinations, there has been significantly less discussion about how end-to-end clinical ultrasound services could benefit from machine learning-based tools. Despite subjectively less AI "hype" in the ultrasound clinical community, the academic outputs grew by more than 600% in the years between 2015 and 2020 (www.pubmed.ncbi.nlm.nih.gov) with the focus now shifting from the basic sciences to clinical translation6. It is becoming more important that clinicians are conversant with data science applications and new technical terms, and are able to critically evaluate the AI literature7,8.
As a diagnostic modality, ultrasound has some key features that have been historically and technologically difficult to address. It is easy to imagine a technology-based solution that would address even a small part of the following US-specific issues:
- Lengthy training processes needed to gain the required ultrasound skills and knowledge.
- Practical skills largely gained directly during clinical examinations (even with some simulation training for entry-level skill development).
- Frequent lone working and variable use of second reviewers.
- Geographic variation in detection rates caused by differences in imaging protocols, training, quality audit and feedback mechanisms.
- Lack of sonographer integration with wider multidisciplinary teams reducing opportunities for appropriate feedback.
- High observer variability and subjectivity in image acquisition, measurements, interpretation and diagnostic reports.
- Limited storage of whole examination imaging data (only limited static images are usually saved).
- Resource intensive quality audit procedures limiting effectiveness.
- Variable and/or relatively frequent changes in equipment and software tools, research and guidance that may impact evidence-based clinical imaging protocols.
- High levels of musculoskeletal (MSK) work-related repetitive strain injury in the workforce.
- Low morale and sustained high levels of vacancies9.
- Potential for medicolegal claims, particularly in obstetric examinations10.
In addition, there will be examples of examination-specific issues where the development of deep-learning-based solutions could improve diagnostic confidence, accuracy or the quality of the service, for example, nodule detection and classification with 2D ultrasound and colour doppler radiomics during thyroid nodule assessment to reduce unnecessary referrals for fine needle aspirations11,12.
Ultrasound as an imaging modality is relatively affordable, portable, safe and available, with a range of clinical applications and increased use by non-radiology-trained healthcare professionals. Given these facts – and the scope to achieve incremental improvements in the holistic ultrasound workflow, workforce and service – it is no wonder this popular imaging modality has received attention from the AI community.
The research landscape
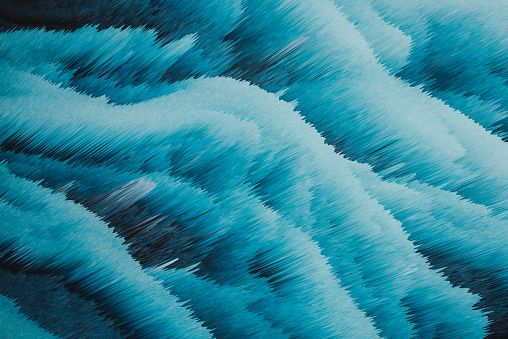
Since AI in radiology, and thus ultrasound, impacts more than solely the diagnostic result contained within the written clinical report, it can be useful to think about the domains in which the ultrasound workflow may be impacted. This could range from:
1. Patient scheduling and preparation. This may include worklist prioritisation, or automated imaging request vetting, using natural language processing or the use of machine-learning algorithms to predict the highest-risk patients using medical history or other clinical data.
2. Standardisation of imaging protocols and acquisition. Automated tools could reduce operator variability and subjectivity or assist novice/unskilled practitioners and include: post-processing tasks such as 3D reconstruction, registration; quality optimisation steps; automated image navigation and guidance; multiple transducer technologies; other advances in 3D imaging methodologies improving its usability.
3. Image and data interpretation. Ultrasound differs from many imaging methods because of the real-time nature of assessment. Ultrasound can provide functional as well as structural data when assessing anatomy and pathology, which could have many applications, including MSK imaging. Automated processes could impact interpretation by providing real-time image capture of high-quality images (Figure 1); automatically assess biometry, potentially with new biometric data inherent to the image, ie radiomics or statistical shape modelling to assess curved or irregular structures; providing image overlays to assist with anatomy identification; or even automatically detect disease and pathology.
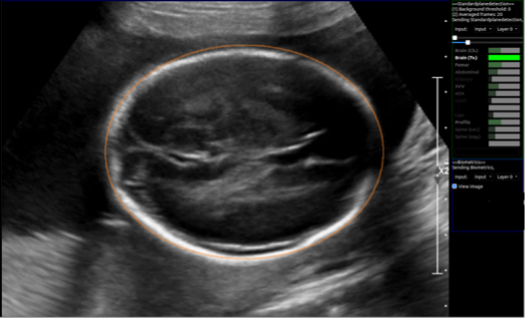
Figure 1. Image of automatic extraction of the fetal head circumference measurement during a real-time scan. The right-hand panel (bright green bar) indicates the confidence that the corresponding ‘brain (Tv)’ plane is correctly visualised. Image courtesy of the iFIND project (www.ifindproject.com)
4. Reporting and recommendations. There is known variability in the way an operator may report findings and there is scope for an AI system to assist in a structured report from data collected automatically during the ultrasound examination, and also to include automated decision support for follow up or disease prediction or prognosis.
That said, as a modality, ultrasound poses some specific engineering challenges that have seen AI development and deployment lag behind other areas of imaging, such as CT, MRI or conventional X-ray12,13. These include the need for large, standardised datasets of complete and routinely collected ultrasound clinical image data (eg. a whole video feed versus selected still images); the need for algorithms that are generalisable between equipment manufacturers; robust handling of variations in image quality; and knowledge of differing local imaging procedures.
In a 2018 consensus meeting, the priorities for AI research were summarised14,15 and include those listed in Table 1. However, there are additional needs related to ultrasound AI tools. Research priorities specific to ultrasound include the need for continued optimisation of 2D and 3D volume sonography (pre-and post-processing), the development of real-time intuitive user guidance, human factors research related to ease of AI tool usage, and generalisability across manufacturer platforms so that algorithms are "vendor agnostic"13. Importantly, real-world adoption of these technologies will rely on appropriate approval procedures and clinical infrastructure – including cloud-based solutions and integration of plug-in-based solutions (Figure 2) – standardisation and, of course, adequate staff training4,13,16,17.
Table 1. Recommendations from the 2018 consensus workshop on translational research held in Bethesda, USA on advancing and integrating artificial intelligence applications in clinical processes (from Weichart et al 2021, adapted from Allen et al 2019, Langlotz et al 2019)13–15
Figure 2. Real time AI-enabled modular software pipeline. The arrows indicate data flow between plug-ins, which are in turn indicated with boxes6 .
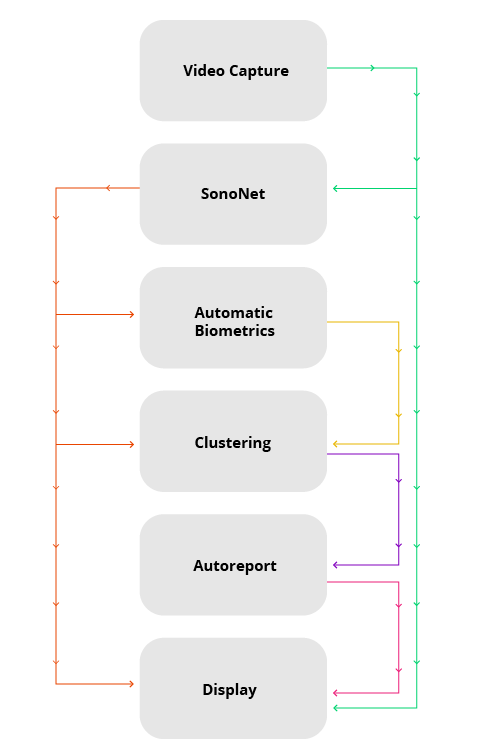
Clinical translation into
ultrasound clinics
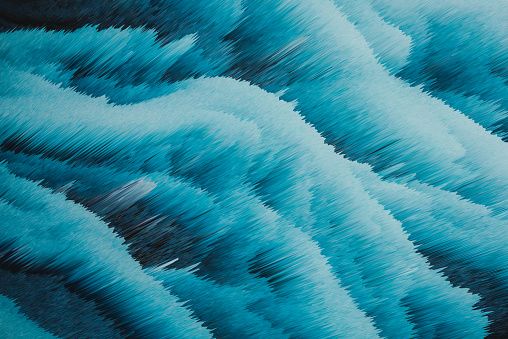
With high levels of activity seen in academia, what can we currently expect to see in our ultrasound machines, and how can we ensure safe implementation and evaluation of these new tools? This is a good question, and overhauls in regulatory frameworks inclusive of specific AI considerations are in progress17. With the interplay of industry commercialisation, academic advancement, regulatory processes, government and public body investment there are complex and multifaceted elements that will impact what ultrasound practitioners will be interacting with in the clinic.
A number of review articles have sought to summarise the individual regulatory-approved AI algorithms intended to be implemented in the ultrasound workflow and the number of ultrasound-related start-up companies in the UK is growing18. Although too numerous to summarise here, applications include AI tools for automated disease classification, object detection within images, image segmentation or prognostic or predictive evaluation2. The clinical applications of include thyroid, prostate or breast nodule assessment aimed at reducing unnecessary biopsies; deep vein thrombosis assessment to support novice clinician interpretation; automated lesion measurement for suspected malignancies of the liver; automatic plane detection and automated biometry extraction, to name a few2,13,19–21.
What is clear is that a collaborative approach is required to answer the most pressing questions so that the deployment of tools with clear value propositions will provide maximal benefit to the services, professionals and patients they are purported to help. We need more tools that can be integrated into the whole ultrasound pipeline. Tools that are less about technology advancement, ie standalone technology-driven solutions, and more about solutions that will solve an important clinical problem and can integrate within existing or redesigned workflows.
Current limitations
and future impact
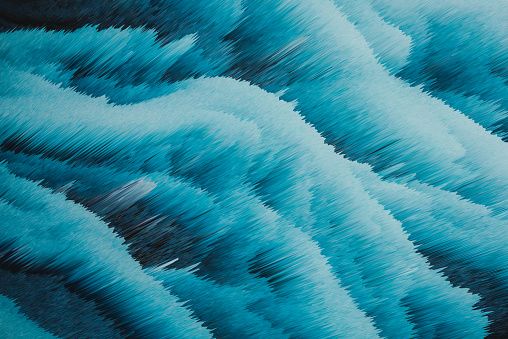
We are currently in the era of "narrow" AI, where specifically developed tools can answer a specifically posed question. Use of tools outside of their intended remit and in the unpredictable real-world setting, as a minimum, will not provide reported performance metrics and, at worst, may offer incorrect and catastrophic clinical decisions. In the BBC's 2021 Reith Lectures1, Professor Stuart Russell suggested that the future of a "general-purpose" super intelligent AI is a reality and it is now urgent that we review how we want to harness this technology for the future.
This is true in the ultrasound domain and, therefore, allowing clinical ultrasound practitioners and managers to harness and deploy the appropriate technology will likely mean learning new skills, adapting professional roles and navigating the impending deluge of novel automated clinical tools and processes that could disrupt the way the workforce and services are organised "before" harm is caused. In this context, harm can be quite nuanced and may include algorithms trained that reinforce or even magnify human biases related to clinical or social inequalities. Harm may mean the adoption of technology that could provide efficiency but at the cost of the human element of care – something that cannot be easily measured but is nonetheless of much value to patients using our services.
Conclusion
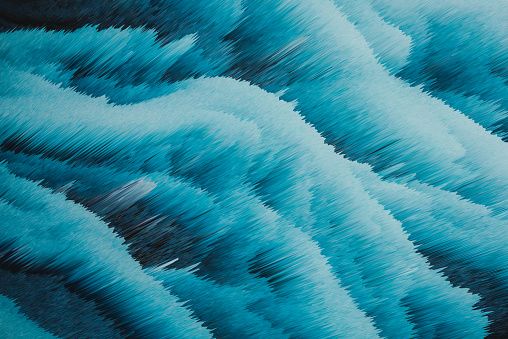
There is no doubt that AI and the related technological advances it will support will inherently impact the way we provide ultrasound-based screening and diagnostic services. The real-time data interpretation and noisy images of this unique modality may mean that the technological development will be slower in its development and adoption – however, there could be much to gain. Ultrasound practitioners supported by technology to reduce operator subjectivity, improve the clinical workflow and increase diagnostic accuracy and confidence could provide more rewarding work, only if coupled with the ability to provide high-quality person-centred care. This will, inevitably, rely on forging strong collaborations with clinical leaders, industry, academia, regulatory bodies and patient stakeholder groups to assure that technology in this field is deployed with the core aim of improving public health and healthcare services.
Jacqueline Matthew MSc, MRes is a Research Sonographer/Radiographer and NIHR Clinical Doctoral Research Fellow (Fetal Imaging) at the Centre for the Developing Brain, Perinatal Imaging and Health Imaging Sciences, the Biomedical Engineering Division at King's College London
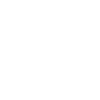
References
1. Prof. Stuart Russell. BBC Radio 4 – The Reith Lectures – Nine Things You Should Know About AI. Available at https://www.bbc.co.uk/programmes/articles/3pVB9hLv8TdGjSdJv4CmYjC/nine-things-you-should-know-about-ai Accessed 4 January 2022.
2. Shen YT, Chen L, Yue WW, et al. Artificial intelligence in ultrasound. Eur J Radiol 2021; 139: 109717.
3. NICE. Artificial intelligence in mammography. Medtech innovation briefing. 2021. Available at www.nice.org.uk/guidance/mib242 Accessed 5 January 2022.
4. Woods T, Ream M, Demestihas M-A, et al. Artificial Intelligence: How to get it right. Putting policy into practice for safe data-driven innovation in health and care.
5. Alexander A, Jiang A, Ferreira C, et al. An intelligent future for medical imaging: a market outlook on artificial intelligence for medical imaging. J Am Coll Radiol 2020; 17: 165–170.
6. Matthew J, Skelton E, Day TG, et al. Exploring a new paradigm for the fetal anomaly ultrasound scan: artificial intelligence in real time. Prenat Diagn. Epub ahead of print 2021. DOI: 10.1002/PD.6059.
7. Kuang M, Hu HT, Li W, et al. Articles that use artificial intelligence for ultrasound: a reader’s guide. Front Oncol 2021; 11: 2062.
8. Drukker L, Noble JA and Papageorghiou AT. Introduction to artificial intelligence in ultrasound imaging in obstetrics and gynecology. Ultrasound Obstet Gynecol. Epub ahead of print 2020. DOI: 10.1002/uog.22122.
9. Centre for Workforce Intelligence. Securing the future workforce supply: sonography workforce supply. 2017. Available at www.cfwi.org.uk Accessed 5 January 2022.
10. Anderson A. Ten years of maternity claims: an analysis of the NHS Litigation Authority data – key findings. Clin Risk 2013; 19: 24–31.
11. Peng S, Liu Y, Lv W, et al. Deep learning-based artificial intelligence model to assist thyroid nodule diagnosis and management: a multicentre diagnostic study. Lancet Digit Heal 2021; 3: e250–e259.
12. Kim YH. Artificial intelligence in medical ultrasonography: driving on an unpaved road. Ultrasonography 2021; 40: 313.
13. Weichert J, Welp A, Scharf JL, et al. The use of artificial intelligence in automation in the fields of gynaecology and obstetrics – an assessment of the state of play. Geburtshilfe Frauenheilkd 2021; 81: 1203–1216.
14. Langlotz CP, Allen B, Erickson BJ, et al. A roadmap for foundational research on artificial intelligence in medical imaging. From the 2018 NIH/RSNA/ACR/The Academy Workshop. Radiology 2019; 291: 781.
15. Allen B, Seltzer SE, Langlotz CP, et al. A road map for translational research on artificial intelligence in medical imaging. From the 2018 National Institutes of Health/RSNA/ACR/The Academy Workshop. J Am Coll Radiol 2019; 16: 1179–1189.
16. Gov.uk. A guide to good practice for digital and data-driven health technologies. Available at https://www.gov.uk/government/publications/code-of-conduct-for-data-driven-health-and-care-technology/initial-code-of-conduct-for-data-driven-health-and-care-technology Accessed 5 January 2022.
17. Gov.uk. Software and AI as a Medical Device Change Programme. Available at https://www.gov.uk/government/publications/software-and-ai-as-a-medical-device-change-programme Accessed 5 January 2022.
18. Signify Research. What’s new for machine learning in medical imaging: Predictions for 2019 and beyond. 2018. Available at https://s3-eu-west2.amazonaws.com/signifyresearch/app/uploads/2018/10/16101114/Signify_AI-in-Medical-Imaging-White-Paper.pdf Accessed 6 January 2022.
19. He F, Wang Y, Xiu Y, et al. Artificial intelligence in prenatal ultrasound diagnosis. Front Med 2021; 0: 2651.
20. Nagendran M, Chen Y, Lovejoy CA, et al. Artificial intelligence versus clinicians: Systematic review of design, reporting standards, and claims of deep learning studies in medical imaging. BMJ 2020; 368: 1–12.
21. Kainz B, Heinrich MP, Makropoulos A, et al. Non-invasive diagnosis of deep vein thrombosis from ultrasound imaging with machine learning. NPI Digit Med 2021 41 2021; 4: 1–18.